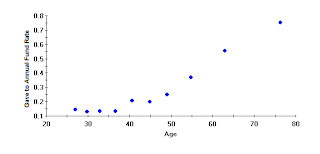
One of the most important things we do during the predictive
modeling process is data preparation, which often means creating new variables
from the data our customers have on-hand. I’d like to discuss some of these
variables, as well as how and why to include them in a fundraising or
advancement model. For the purposes of this blog entry, I’ll use a higher
education example. Typically a higher education institution might have some
extra variables, but these can be tailored to fit other institutions or
excluded when not relevant.
Demographic
Information
It’s always smart to have an idea of what each donor looks
like at a demographic level. Variables to include here are things like age,
gender, marital status, and any occupational data you might have available to
you. In a higher education context, this would also include things like the
constituent’s class year, major, whether or not their spouse is an alumni, and
whether they are a legacy alumni (meaning a parent or grandparent also attended
the institution). Additionally, we often create a “reunion year flag”
indicating if the analysis year is a reunion year for that person, as donors
are often more likely to give (and give larger gifts) during a reunion year.
Location Information
General information about each donor’s location like ZIP
code, city, and county can be useful as categorical variables (treating people
that live in each one as a group). Once we have a ZIP code, we always calculate
a “distance from institution” variable using one of Veera’s pre-programmed
functions. This new variable, which is measured in miles, gives you a solid
idea of the relationship between location and giving. If you have access to
census data, we recommend appending variables relating to neighborhood or
housing type. Creating flag variables for wealthy neighborhood ZIP codes can
also be useful; constituents coming from these areas may be more likely to give.
Although this can be created at a more local level, we often start with Forbes’
list of the top 500 wealthiest ZIP codes in the US, which is available online
at http://www.forbes.com/lists/2011/7/zip-codes-11_rank.html.
Contact History
The ways in which a donor engages with you can tell you a
lot about their likelihood of giving. For starters, include variables
pertaining to their event history. How many events have they attended? Which
types of events are they attending? How many days since their last event?
Answers to questions like these can sometimes turn out to be predictive of
giving. This is also where your social media variables come into play; create
flags for whether a constituent is following you on LinkedIn, Facebook,
Twitter, Pinterest, etc. A donor following you on one or more of these sites is
an indication that they want to be connected, and therefore they may be more
likely to give. Conversely, if a constituent has indicated that they do not
want to be contacted, you’ll want to include this information as well, as it
can be very predictive.
Gift History
This brings us to our last and most predictive set of
variables: giving history. These variables should answer all kinds of questions
about what a giver looks like historically, like:
- How many gifts have they given in their lifetime?
- What was their last gift?
- How many days since their first gift? How many days since their last gift?
- Have they given in the past 12 months? If so, how much?
- What is the velocity of the gifts - are the increasing, decreasing, or staying the same?
One thing to note here is that gift dates themselves aren’t
useful in a predictive model, but their translations – like the number of days since an event – allow us to use the insight they provide.
In building a predictive model, some of these variables may
be predictive, while others might turn out not to be. It’s a good idea to
include some combination of these variables, plus anything you have on-hand
that you think could possibly be predictive.
-Caitlin Garrett, Statistical Analyst at Rapid Insight
-Caitlin Garrett, Statistical Analyst at Rapid Insight
A few things I would add, mostly random thoughts:
ReplyDeleteparticipation on athletic teams and clubs, and other extra curricular activities provide insight on the student's involvement which can translate to the alumni's involvement
Recency, frequency, and monetary value of past gifts and pledges are the bywords for prediction
If a reply card was used in the past, the marketing material used becomes a part of the pledge and giving record, and should be an indicator for the next giving contact.
Commercial lists of zip codes and market areas defines who to invite to an off-campus event (this is more an operational consideration based on a predictive analysis)
Economic history generally impacts giving and pledges and economic forecasts should be in the predictive model, which belies the idea of ignoring gift dates (contact your Federal Reserve or economics department)
Some campaign dates are geared around the holidays for a good reason.
In capital campaigns, the campaign for a science center takes on different characteristics from a campaign for a stadium, and an endowment campaign has different model values from the first two.
A professor who taught a memorable student may have an opinion about the student's generosity as a boolean or a quantifiable data type.
The alumni or development officer past success should be an indicator of future success.
Your mileage may vary and if you are not using a statistician, you should be.
My apologies for the delay in responding to this - this list contains some really great ideas for new variables.
ReplyDeleteTracking marketing efforts and any changes made in the way in which a constituent is marketed to can be especially helpful.Creating a "holiday" flag for mailings that come during that time of year could also be very predictive.
Good suggestions!