Establish your goals
This first step is crucial for mapping out a course to your
end goal. Try to envision where you’d like to end up and formulate a specific goal
to help get you there.
Possible goals include:
- Reduce your prospect mailing budget
- Increase accuracy of enrollment yield predictions
- Meet diversity objectives
- Increase your retention rate
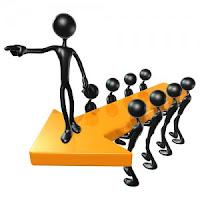
Get to know your data
The first step to getting to know your data is gaining
access to your data, which is trickier for some people than others. If you have
to go through IT to access your data, it helps to have a clear goal in mind and
a good idea of what fields or tables you’ll need.
Once you have your data, you’ll need some time to get
well-acquainted. A good starting point is to make sure you understand what each
field represents and how things are coded. If you have questions about how data
is being recorded or stored, this is the time to ask. Once you have a handle on
what your data represents, you’ll want to thoroughly review it.
A few suggestions:
- Spot-check the accuracy of your data. Double-checking
things like the mean, min, and max for each variable is a quick way to verify
accuracy. If you spot any data quality
issues, do your best to resolve them sooner than later.
- Check for missing values. If you have a variable with a high number of missings, you’ll need to decide whether or not to use that variable and if there’s a way to fill in what’s not there.
- Brainstorm ideas for new variables. If you can’t create new variables from what you have on-hand, spend some time thinking about things that might be worth tracking going forward.
Analyze your data
I realize that the word “analyze” represents a whole
spectrum of techniques and applications – and that’s okay. In a general sense,
you’ll want to see if fields in your dataset can give you some insight that you
can relate back to your initial goal(s).
Some ideas:
- Look at correlations within your dataset. Are they positive or negative? Large or small?
- Look for the differences between your target and non-target population, variable by variable.
- Visuals help! Graphs are a great way to get a feel for the relationships between your variables.
- Try building a predictive model. The results you get will be more directly applicable to driving decisions.
You may get some surprising results during the analysis
phase. I’ve worked on projects where the end insight was the exact opposite of
what was expected. Although sometimes the results can be surprising, it’s
important to let your data tell its story. The other side of analysis is that
your data can confirm what you’ve long-suspected to be the truth – whether it’s
that students from Montana are more likely to enroll, or that the number of
first term credits impacts a student’s likelihood of attrition – embrace these
confirmations and continue to rely on that information.
Turn analysis into
insight
Keep your initial question in mind, take what you’ve learned
from your analysis, and apply it going forward. The idea here is to replace
outdated anecdotal evidence with insights from our data. If your goal was to
save money on prospect marketing efforts, use the factors that correlate to a
higher response rate to drive your decisions about who will receive the next
round of direct mail. If you’re trying to improve retention rate, target those
students who look most like previously dropped students and reach out to help
keep them on campus.
Assess your decisions
Last, but certainly not least, don’t forget to circle back
and re-assess your decisions. If you feel like you’re not making progress
toward your initial goal, consider re-framing it or breaking it down into more
manageable phases. If you feel good about the progress you’re making, start
working on new goals. A data-driven decision should be sustainable under
conditions similar to the past. Don’t be afraid to revisit past goals if you
feel like you can improve or add something to your initial recommendation.
...Did we miss anything? Have questions about becoming more data-driven? Leave them in the comments below.
...Did we miss anything? Have questions about becoming more data-driven? Leave them in the comments below.
-Caitlin Garrett, Statistical Analyst at Rapid Insight
No comments:
Post a Comment